
Photographic digital image analysis (DIA) is frequently used in seral, single-strata vegetation, given its greater objectivity and repeatability compared to observer visual estimation however, its efficacy in multi-strata forest vegetation may be compromised, where various visual obstructions (coarse downed wood-CDW-conifer regeneration, and shadows) may conceal plant cover in the digital imagery. Reliable estimates of understory (non-tree) plant cover following fire are essential to assess early forest community recovery. The results obtained indicate that the color information of 3D point clouds is an alternative to support individualizing trees under conditions of high-density vegetation.
#HDRSOFT PHOTOMATIX PRO V5.0.5 MANUAL#
The results were evaluated by contrasting them with results of manual individual tree identification on the UAV orthomosaic and those obtained by applying a progressive triangulated irregular network to the 3D point cloud. Subsequently, an automatic crown identification procedure was applied to the CHM. Then, a CVI was applied to 3D point cloud to differentiate between vegetation and nonvegetation classes to obtain a DEM and a CHM. UAV flights were carried out, and a 3D dense point cloud and an orthomosaic were obtained.

The objective of this study was to evaluate the use of color vegetation indices (CVI) in canopy individualization processes of Pinus radiata. Although the results are satisfactory, the quality of this detection is reduced if the working area has a high density of vegetation. From this DEM, a canopy height model (CHM) is derived for individual tree identification. A dense 3D point cloud is generated from RGB UAV images, which is used to obtain a digital elevation model (DEM). The very high spatial resolution of UAV imagery supports these processes. The location of trees and the individualization of their canopies are important parameters to estimate diameter, height, and biomass, among other variables. The obtained results show that the use of HDR images improves the detection performance to more than 30% when compared to LDR. In order to prove their advantage in image processing, a comparative analysis between LDR and HDR images, for fruits detection and counting, was carried out.
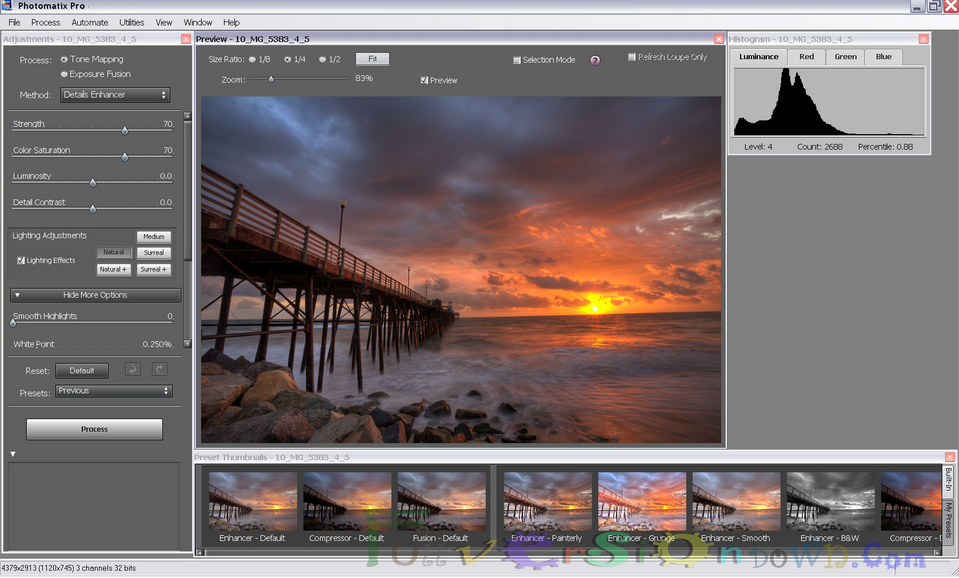
However, this issue can be handled by High Dynamic Range (HDR) images since they have the ability to store luminance information similarly to the human visual system. Real scenes, observed in natural environment, present high dynamic ranges that cannot be represented by the common LDR (Low Dynamic Range) devices. As such, accurate representation of the gathered production images is a major concern, especially if those images are used in detection and classification tasks.
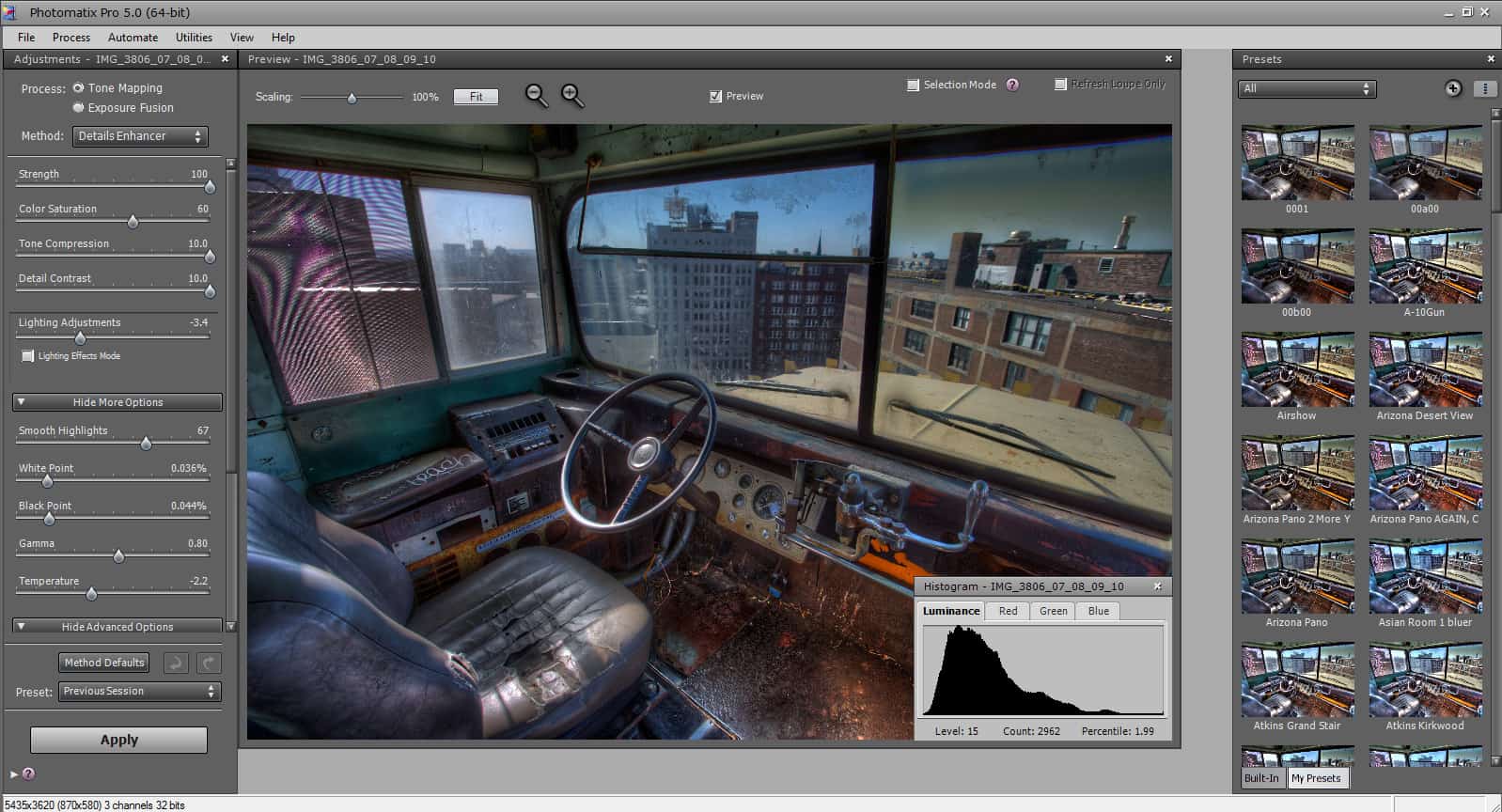
In this framework, imaging techniques that provide visual information over the farming area play an important role in production status monitoring. This new production concept relies on the use of information technology (IT) to provide a control and supervising structure that can lead to better management policies. Precision agriculture is gaining an increasing interest in the current farming paradigm. We conclude that HDR imagery can provide more accurate measurements of bare soil cover for ecosystem monitoring and assessment. Drawbacks to the method included decreased image sharpness due to minor misalignment of images or moving vegetation, time required to create HDR images, and difficulty with acquiring primary images from a moving platform. HDR images showed more detail reduced the numbers of pure black, pure white, and pixels visually indistinguishable from black and white reapportioned skewed luma values towards a normal distribution and increased the Euclidean distance between litter and bare ground RGB values-allowing increased feature separation all of which facilitated an increase in real feature classification through manual image analysis. HDR composites were created by merging three differentially exposed images spanning a wide exposure range and resulted in lightened shadows. To mitigate shadow effects in near-earth imagery (2 m above ground level), we created high dynamic range (HDR) nadir images and used them to measure grassland ground cover. Shadow often interferes with accurate image analysis.
